Minimally invasive surgical robots, such as the Intuitive Surgical da Vinci robot, allow surgeons to remotely operate surgical tools through incisions smaller than the width of a human finger. While these telerobotic laparoscopic systems allow for greater dexterity and improved vision over traditional laparoscopic surgery, they do not allow the surgeon to feel the interactions between the robot and the surgical environment. Experienced robotic surgeons have, therefore, learned to heavily rely on vision to guide their manipulations with the robot and estimate physical properties of tissue. For novice surgeons, this skill can only be developed with repeated practice and training. Still, vision is not always as accurate as the sense of touch, as demonstrated by my work on prosthetics. Thus, there exist a number of surgical procedures in which the surgeon needs to palpate or “feel” the tissue to determine critical information, such as the location of blood-carrying vessels or the boundaries of a cancerous tumor. My work in robotic minimally invasive surgery (RMIS) has two complimentary foci: investigating strategies for advanced training platforms for RMIS and investigating strategies for pinching palpation feedback in RMIS.
Advanced training platforms for RMIS
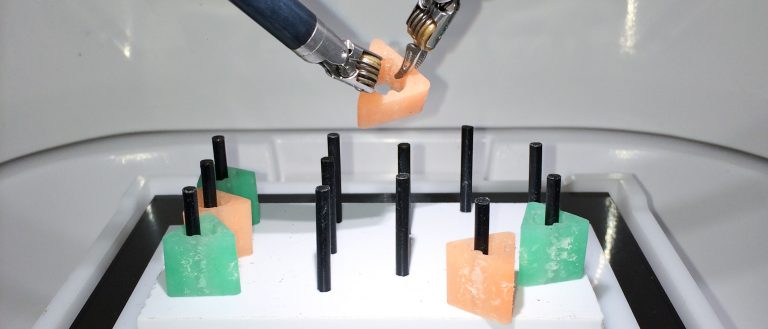
For a novice surgical trainee, learning the technical skills needed to effectively and efficiently operate a minimally invasive surgical robot can be particularly daunting. Many trainees spend considerable time practicing inanimate training tasks with the clinical robot to develop the necessary technical skills. Training with the clinical robot, however, requires the use of expert human graders to evaluate a trainee’s performance. This evaluation process can be subjective, time-consuming, and cost-ineffective as most experts are practicing physicians themselves.
To address this challenge, I am investigating the use of advanced training platforms that measure the physical interactions between the surgical robot and the training environment to access a trainee’s skill at a given inanimate training task. The current system, the Smart Task Board, uses the task completion time, high frequency accelerations of the two primary robotic tools and the robotic camera, as well as the forces produced on the training task to evaluate skill according to a standardized assessment tool. To accomplish this, machine learning models are trained to recognize patterns between the time, acceleration, and force data, and ground truth labels of skill provided by expert human graders.
A key drawback of robotic surgery platforms is that they do not provide haptic cues. This makes it difficult for surgeons to learn to operate, as they must learn to rely on their eyesight to characterize cues normally detected by touch, such as force. Substantial research has investigated the incorporation of haptic feedback into surgery platforms, but less research has been done to learn about how haptics can be used to train surgeons for robotic surgery. By providing users with force feedback during training, we predict that they will more rapidly learn to operate with only their eyes. Surgeons experienced in robotic surgery often say that they have learned to “feel with their eyes.” We believe that haptics can teach this skill more effectively.
[1] J. D. Brown, C. E. O’Brien, K. W. Miyasaka, K. R. Dumon, and K. J. Kuchenbecker, “Analysis of the Instrument Vibrations and Contact Forces Caused by an Expert Robotic Surgeon Doing FRS Tasks,” in 8th Annual Hamlyn Symposium on Medical Robotics, 2015, pp. 75–77.
[2] J. D. Brown, C. E. O’Brien, S. C. Leung, K. R. Dumon, D. I. Lee, and K. J. Kuchenbecker, “Using Physical Interaction Data of Peg Transfer Task to Automatically Rate Trainee’s Skill in Robotic Surgery.,” Under review for IEEE Transactions on Biomedical Engineering.
Read More